Introduction
HQS Quantum Solver enables you to run precise simulations of quantum systems by accounting for all interactions between the particles in a system.
HQS Quantum Solver gives you easy access to high-performance routines and solvers needed to work with quantum systems.
Providing a consistent and extensible interface, it offers routines for the construction of Hamiltonians and operators in either full configurational space or subspaces characterized by conserved quantities such as the spin or the particle number.
Interested in high-quality time evolution? We offer an optimized Krylov implementation of the time-evolution propagator. By using Krylov methods, we target exactly the space of interest and preserve the unitarity of the wavefunction at a fraction of the cost of conventional methods. See the theory section for more details.
In addition to providing a framework, multiple backends have been implemented for the efficient evaluation of properties depending on the user's needs.
We provide a consistent protocol allowing for user-driven extensibility; users can write their own backends, tools, and algorithms and interface with ours.
Installation
HQS Quantum Solver
Visit cloud.quantumsimulations.de/software, search for "HQS Quantum Solver" in the package list and select "Download". Follow the instructions on that page to install HQS Quantum Solver.
To use HQS Quantum Solver, you furthermore need to install the Intel Math Kernel Library (MKL), which is discussed in the next section.
MKL
If you want, you can manually provide a version of the MKL by making sure
that the file libmkl_rt.so
is found by the dynamic linker. That means,
that a system-wide installation should be found automatically.
If you use HQStage to install HQS Quantum Solver, you can install the MKL into an HQStage managed environment by running the following command.
hqstage envs install-mkl
When using your own virtual environment you can install the MKL via pip.
However, you need to create a symlink for the file libmkl_rt.so
. The
commands below perform the necessary steps.
pip install mkl
PLATLIB_DIR="$(python -c "import sysconfig; print(sysconfig.get_path('platlib'))")"
ln -fs libmkl_rt.so.2 "${PLATLIB_DIR}/../../libmkl_rt.so"
Getting Started
Since quantum mechanical systems are described by operators acting on state vectors living in Hilbert spaces, this library is mainly concerned with providing such operators and functions that manipulate state vectors. In this chapter, you will learn the essentials of using the HQS Quantum Solver software by considering an example computation.
Let us consider the tight-binding Hamiltonian defined on a chain lattice, i.e., the Hamiltonian is given by where is the number of lattice sites, the strength of the hopping term and the annihilation operator at site .
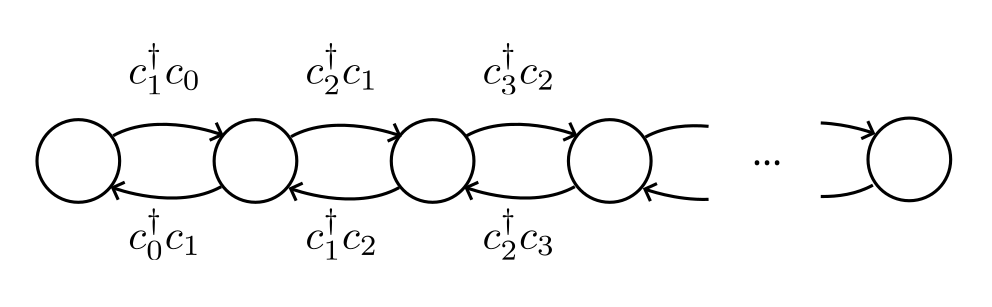
Imports and Parameters
First, we need to import the functions, classes and modules that are required for the script. If you want to follow along, copy the following imports into your script file. We will explain the classes and functions as we go along.
# Title : HQS Quantum Solver Getting Started Guide
# Filename : getting_started.py
# Python, NumPy and SciPy imports
from math import pi, sqrt
import numpy as np
from scipy.linalg import toeplitz
from scipy.sparse.linalg import eigsh
import matplotlib.pyplot as plt
# HQS Quantum Solver imports
from hqs_quantum_solver.operators import (
SpinlessFermionOperatorEigen as SpinlessFermionOperator
)
from hqs_quantum_solver.types import (
SpinlessFermionHamiltonianInput,
SpinlessFermionAnnihilationInput,
)
from hqs_quantum_solver.interface import scipy_operator_adapter
Next, we define the parameters that we will refer to in the script.
M = 16 # site count
N = 1 # particle count
E = 0 # energy level
With that out of the way, we can start setting up the tight-binding Hamiltonian.
Setting up the Hamiltonian
We want to look at a system given by the tight-binding Hamiltonian. Thus, the first step is to construct said Hamiltonian. Quantum Solver provides a generic interface for constructing Hamiltonians, as well as several convenience functions. Especially, Quantum Solver implements Hamiltonians of the form where is the, so called, hopping matrix. Note, that the additional terms default to zero unless we explicitly enable them.
If we define the matrix to be then the Hamiltonian in the previous formula becomes the tight-binding Hamiltonian defined at the beginning of this chapter.
This matrix can be easily constructed using the
toeplitz
function from SciPy.
t = 1
c = np.array([-t if j == 1 else 0 for j in range(M)])
h0 = toeplitz(c)
With this matrix at hand, we can construct the Hamiltonian.
Quantum Solver supports multiple linear algebra backends. For this reason,
the construction of quantum mechanical operators is a two-step process.
First, we create an "Input" object that describes the operator to be
constructed. Then, we pass this object to an operator class of the desired
backend. Note that we have imported the
SpinlessFermionOperatorEigen
as SpinlessFermionOperator
, which means that
we are using the Eigen backend, which implements its functionality using
the well-known Eigen library.
To create the "Input", we create a
SpinlessFermionHamiltonianInput
object. The constructor of this object expects
four requried arguments and a bunch of additional arguments. The additional
arguments define the additional terms mentioned above. The required arguments
are M (int)
, N (int)
, mod_N (int)
, and h0 (ndarray)
.
The parameter M
describes the number of sites in the system and
the parameter N
the number of particles in the system.
The parameter mod_N
sets the step size in which the number of particles
is allowed to change during the simulation. For example for
M = 10
, N = 5
and mod_N = 4
the system allows for states with
1, 5, and 9 particles. When mod_N = 0
the number of particles is conserved,
i.e., constant.
The parameter h0
is the "hopping matrix" given as a NumPy array.
With all this information, we can create the Hamiltonian by first creating the "Input" object and then handing it over to the linear algebra backend.
H_input = SpinlessFermionHamiltonianInput(M=M, N=N, mod_N=0, h0=h0)
H = SpinlessFermionOperator(H_input)
The Quantum Solver operators
primarily provide matrix-vector and matrix-matrix
routines.
Here, we will be using the
SpinlessFermionOperatorEigen
.
As a first example for using the
operator, we want to look at its spectrum. For that, we can use
the eigsh
function from SciPy. To use this function, however, we need to use an
adapter
(see scipy_operator_adapter
)
to make the Quantum Solver operator compatible with SciPy.
k = min(12, H.shape[0] - 1) # Number of eigenvalue to compute
evals, evecs = eigsh(scipy_operator_adapter(H), k=k, which="SA")
print(evals)
plt.figure("eigenvalues", clear=True)
plt.title(f"Eigenvalues (M = {M}, N = {N})")
plt.plot(evals, ".")
Now, we have enough code to run the script for the first time. Doing so should produce the plot shown below, which shows the ten smallest eigenvalues of the Hamiltonian, and hence, the lowest energy levels of the system.
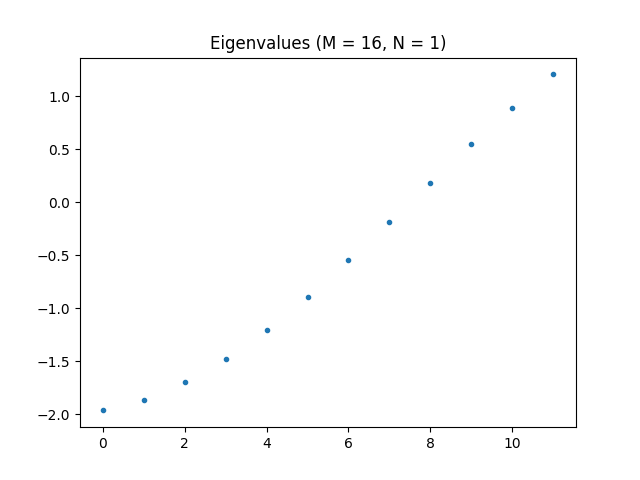
Before continuing, modify the values of and , run the script, and look at the result a few times.
Note, for mod_N=0
the number of states Quantum Solver has to store is
. Hence, the number of states can grow rather quickly when
increasing N
while M
is large. This problem gets worse for mod_N != 0
.
Keep in mind, while
Quantum Solver provides you with highly accurate computations, you will
have to provide enough computational resources for it to shine.
Below, we have listed the 5 smallest eigenvalues for M = 16
and different
values of N
.
# | |||
---|---|---|---|
1 | -1.97 | -3.83 | -5.53 |
2 | -1.86 | -3.67 | -5.31 |
3 | -1.70 | -3.57 | -5.14 |
4 | -1.48 | -3.44 | -5.04 |
5 | -1.21 | -3.34 | -5.04 |
We can make our first observation: Adding the first two values of the column gives you the first value in the column, and adding the first three values of the column will give you the first value in the column. The reason for this is that in a two- and three-particle system, the lowest energy to obtain is the one of the particles occupying the lowest two and three energy levels, respectively. Since we are dealing with a fermionic system, the particles are not allowed to occupy the same state, which explains this behavior.
We shall later come back to this observation.
Note how few lines of code were necessary, to produce physically meaningful results.
Site Occupations
To take this example a little bit further, let us look at the computation of site occupation expectation values. More precisely, we want to compute for , where . Note that Hence, we just need to compute and then compute the square norm of this vector.
First, we have to construct the annihilation operator .
As before, we first have to create an input object that we then hand over
to the linear algebra backend.
This time we create an SpinlessFermionAnnihilationInput
object.
The constructor expects the parameters
M (int)
, N (int)
, and mod_N (int)
, which
describe the domain, i.e., the input, of the operator and have the same meaning as
before. Additionally, we need to provide an argument called
c_i (int | ndarray)
, which describes which annihilation operator is to be
constructed. When passing an integer j
, the annihilation operator for site
j
is constructed.
(See SpinlessFermionAnnihilationInput
.)
Note that the codomain of the operator has N - 1
particles, so the
operator maps to a different space.
Once we have constructed the operator, we can use the
dot
method, to apply it to a state vector.
Below, we define a function site_occupation
which computes the expectation
value for a given state vector and site. We then plot the expectation
value for every site and for the state vector corresponding to the
energy level E
.
def site_occupation(psi, j):
annihilation_input = SpinlessFermionAnnihilationInput(M=M, N=N, mod_N=0, c_i=j)
annihilation = SpinlessFermionOperator(annihilation_input)
c_psi = annihilation.dot(psi)
return c_psi.conj() @ c_psi
occupations = np.array([site_occupation(evecs[:, E], j) for j in range(M)])
plt.figure("site_occupation", clear=True)
plt.title(f"Site Occupation (M = {M}, N = {N}, E = {E}")
plt.plot(occupations, "x-")
plt.axis((0, M - 1, 0, 1))
plt.xlabel("j")
plt.ylabel("Expectation Value")
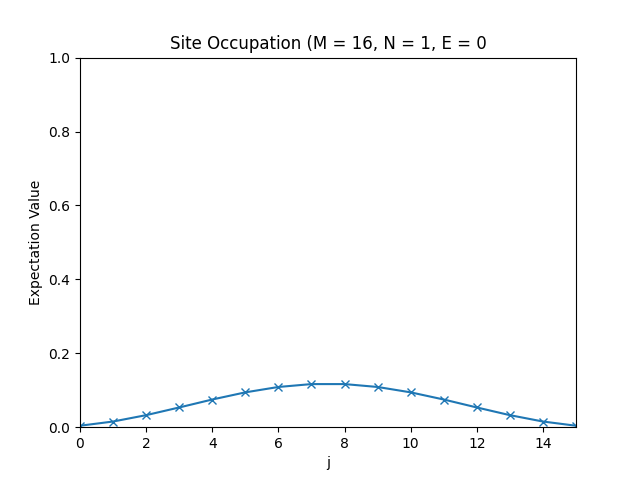
The site occupations for M=16
, N=1
, E=0
.
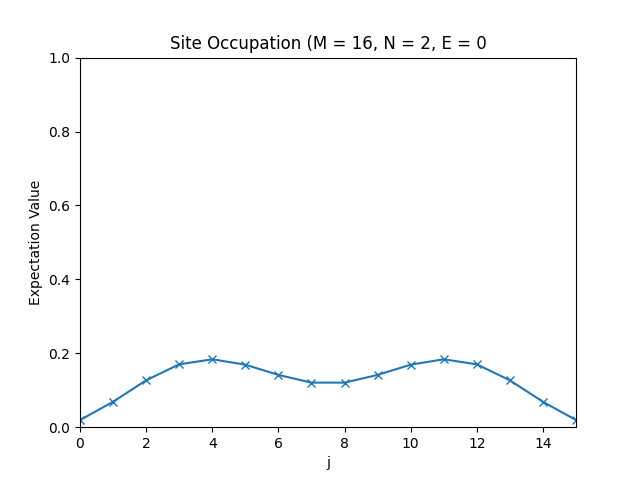
The site occupations for M=16
, N=2
, E=0
.
Energy occupations
While looking a site occupations is certainly interesting, for most applications it is more useful to look at energy occupations instead.
Consider the matrix given by The columns of this matrix are the eigenvectors of the matrix . It is a well-known fact that if we define we can write the Hamiltonian as where are the energy levels of the system. Consequently, we can measure expectation value of the occupation of the -th energy level, by computing
We can carry out this computation in a similar way to the computation of
the site occupation. All we have to do is to set the annihilation
variable
to a linear combination of annihilation operators, which is given by the
definition of .
Luckily, this is such a common operation that the Quantum Solver includes a
convenience method for exactly this case.
The c_i
parameter of the constructor of the
SpinlessFermionAnnihilationInput
also accepts an array of coefficients and creates the corresponding
linear combination of annihilation operators.
J, K = np.meshgrid(np.arange(M), np.arange(M), indexing='ij')
Q = sqrt(2) / sqrt(M+1) * np.sin((J+1) * (K+1) * pi / (M+1))
def energy_occupation(psi, k):
annihilation_input = SpinlessFermionAnnihilationInput(
M=M, N=N, mod_N=0, c_i=Q[:,k].conj()
)
annihilation = SpinlessFermionOperator(annihilation_input)
c_psi = annihilation.dot(psi)
return (c_psi.conj() @ c_psi).real
ks = np.arange(M)
expectation = np.array([energy_occupation(evecs[:, E], k) for k in ks])
plt.figure("energy", clear=True)
plt.title(f"Energy Occupation (M = {M}, N = {N}, E = {E})")
plt.plot(ks, expectation, "x-")
plt.xlabel("p")
plt.ylabel("Expectation Value")
Below, you can find the plots that this script produces for different parameter configurations.
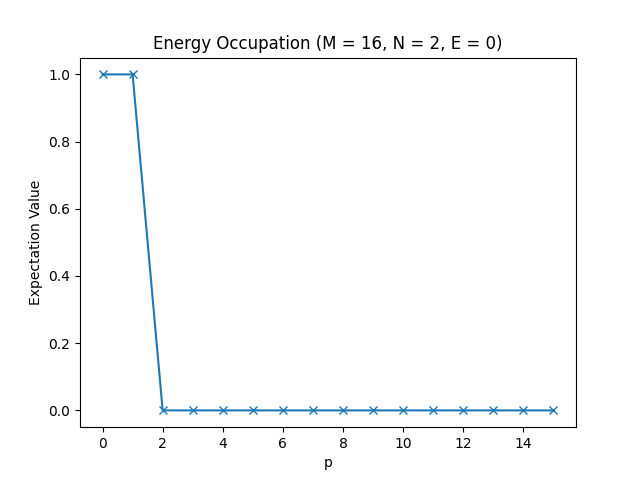
The energy occupations for M=16
, N=2
, E=0
.
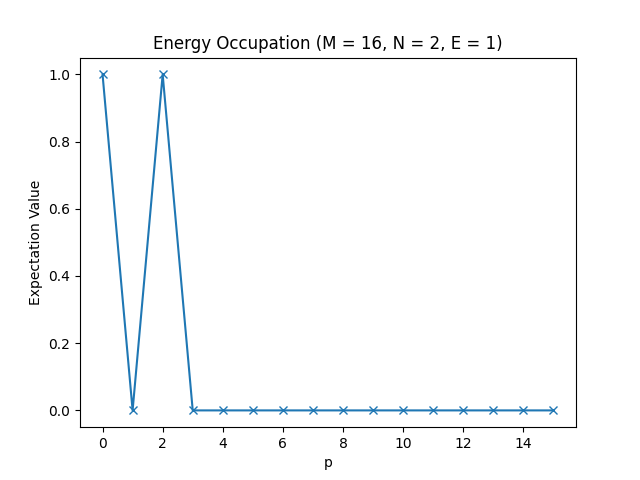
The energy occupations for M=16
, N=2
, E=1
.
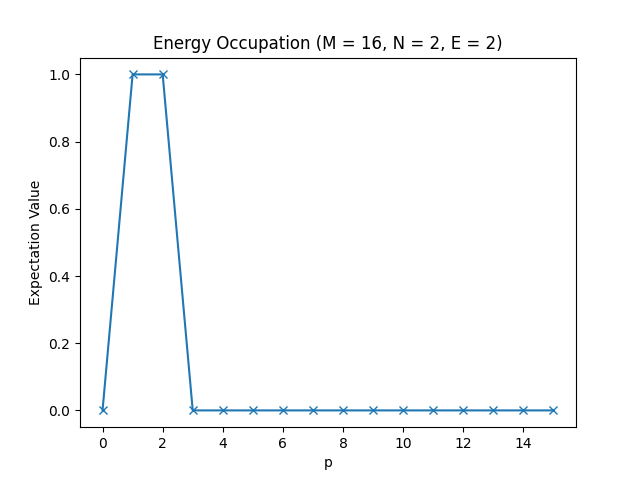
The energy occupations for M=16
, N=2
, E=2
.
In these figures, you can see how the fermions arrange in the different one-particle energy states to achieve the energy levels we have observed in the table in the Setting up the Hamiltonian section.
Note that if you do not know the eigenvectors of the matrix , you can
easily obtain them by using the
eigh
function of NumPy.
Summary
To conclude this introduction, let us sum up the main take-aways of this chapter.
- Quantum Solver works by providing classes for working with quantum mechanical operators.
- Operators are created by constructing an "Input" object and handing it to a linear algebra backend.
- The parameters
M
,N
,mod_N
describe the domain of the operator, whereM
is the number of sites,N
the number of particles, andmod_N
the step size by which the number of particles is allowed to change. - To convert Quantum Solver operators into SciPy linear operators, you can
use the
scipy_operator_adapter
function. - Operators provide the
dot
method, which applies the operator to a state vector.
Complete Code
# Title : HQS Quantum Solver Getting Started Guide
# Filename : getting_started.py
# Python, NumPy and SciPy imports
from math import pi, sqrt
import numpy as np
from scipy.linalg import toeplitz
from scipy.sparse.linalg import eigsh
import matplotlib.pyplot as plt
# HQS Quantum Solver imports
from hqs_quantum_solver.operators import (
SpinlessFermionOperatorEigen as SpinlessFermionOperator
)
from hqs_quantum_solver.types import (
SpinlessFermionHamiltonianInput,
SpinlessFermionAnnihilationInput,
)
from hqs_quantum_solver.interface import scipy_operator_adapter
# ===== Parameters =====
M = 16 # site count
N = 1 # particle count
E = 0 # energy level
# ===== Creation of the Hamiltonian =====
t = 1
c = np.array([-t if j == 1 else 0 for j in range(M)])
h0 = toeplitz(c)
H_input = SpinlessFermionHamiltonianInput(M=M, N=N, mod_N=0, h0=h0)
H = SpinlessFermionOperator(H_input)
# ===== Computing the Eigenvalues and Eigenvectors =====
k = min(12, H.shape[0] - 1) # Number of eigenvalue to compute
evals, evecs = eigsh(scipy_operator_adapter(H), k=k, which="SA")
print(evals)
plt.figure("eigenvalues", clear=True)
plt.title(f"Eigenvalues (M = {M}, N = {N})")
plt.plot(evals, ".")
# ===== Plotting the Site Occupations =====
def site_occupation(psi, j):
annihilation_input = SpinlessFermionAnnihilationInput(M=M, N=N, mod_N=0, c_i=j)
annihilation = SpinlessFermionOperator(annihilation_input)
c_psi = annihilation.dot(psi)
return c_psi.conj() @ c_psi
occupations = np.array([site_occupation(evecs[:, E], j) for j in range(M)])
plt.figure("site_occupation", clear=True)
plt.title(f"Site Occupation (M = {M}, N = {N}, E = {E}")
plt.plot(occupations, "x-")
plt.axis((0, M - 1, 0, 1))
plt.xlabel("j")
plt.ylabel("Expectation Value")
# ===== Plotting the Energy Occupations =====
J, K = np.meshgrid(np.arange(M), np.arange(M), indexing='ij')
Q = sqrt(2) / sqrt(M+1) * np.sin((J+1) * (K+1) * pi / (M+1))
def energy_occupation(psi, k):
annihilation_input = SpinlessFermionAnnihilationInput(
M=M, N=N, mod_N=0, c_i=Q[:,k].conj()
)
annihilation = SpinlessFermionOperator(annihilation_input)
c_psi = annihilation.dot(psi)
return (c_psi.conj() @ c_psi).real
ks = np.arange(M)
expectation = np.array([energy_occupation(evecs[:, E], k) for k in ks])
plt.figure("energy", clear=True)
plt.title(f"Energy Occupation (M = {M}, N = {N}, E = {E})")
plt.plot(ks, expectation, "x-")
plt.xlabel("p")
plt.ylabel("Expectation Value")
Examples
Before looking at the examples, it is advised that you read the Getting Started Guide.
The examples described below can be accessed via HQStage.
-
spinful_fermions_groundstate.ipynb
In this example, we show how to compute the ground state of the Hamiltonian of a many-particle system.
-
spin_waves.ipynb
This example introduces spin systems and time evolution. We consider the time evolution of a Heisenberg spin model in an excited state.
-
nonequilibrium_electron_transport.ipynb
This example simulates the time evolution of a system of electrons starting in a nonequilibrium state.
-
spinless_fermions_greens_function.ipynb
Example for the Calculation of the Spectral function using HQS Quantum Solver and Lattice Functions.
This method combines operators, Chebyshev expansion, cluster perturbation theory and clever Green's function mathematics to create the reciprocal-space resolved spectral function (a. k. a. the interacting band structure) in a honeycomb lattice causing an opening of the Dirac point.
-
spinful_fermions_greens_function.ipynb
Prototype example for the Calculation of the Spectral function of the Hubbard model using hqs_quantum_solver. In this approach we represent the wavefunction as a dyadic product of up and down states.
Similar to the previous example, this example computes the interacting band structure of a square lattice under the influence of interaction. We retrieve the expected behaviour of opening a gap at half-filling.
-
lidar.ipynb
In this scientific example we study properties of light emitted by multiple atoms, a fundamental model in quantum optics. We use the HQS Quantum Solver to time evolve the system according to the Lindblad equation of motion and calculate various expectation values of system operators describing properties of the emitted light.
Reference
You can find the API reference here.
Theory
Time Evolution using Krylov Methods
In this section, we will discuss some of the mathematics behind time-evolution using Krylov methods.
First, a refresher on a Krylov subspace. Given an initial state , and matrix , the order Krylov subspace is given by:
where span refers to the space spanned by the vectors. In practice, this list of vectors is converted into an orthonormal basis by (modified-)Gram-Schmidt methods, Givens rotations or Householder reflections.
For the evaluation of the time-propagator, we seek approximations of the form:
that is, that we can expand the effect of our evolution operator as a polynomial of order (in ). To do so, first we define our orthonormal basis using the Arnoldi method:
compute for : compute for in :
then our basis is comprised of the vectors and the subscript refers to the fact that this is the basis formed from the order Krylov subspace.
The Arnoldi method has the useful property, that upon applying the basis vectors to the original matrix, the resulting smaller matrix , is tridiagonal when is Hermitian, and is upper-Hessenberg otherwise. Both are more easily exponentiated, but the tridiagonal case especially so.
Now that we have our basis, we seek to find an approximate solution . More precisely, we are looking for a solution of the minimization problem
Since the columns of are orthonormal, we can split the identity into the projection onto the range of and its orthogonal complement via . Thus,
Expanding, using the pythagorean theorem, and simplifying gives
Note, that the second norm on the right does not include the variable , while choosing
makes the first norm on the right equal to zero. Since norms are non-negative, this expression is, therefore, the desired minimizing argument .
This equation, however, still involves the original matrix exponential. By noting in the orthogonalization process of the Krylov subspace, that ( being the unit vector at index ), we rewrite our solution as
Hence
We would like to move the matrice into the exponentiation, because then we would be able to compute by
which only involves the small matrix . However,
since . Let us assume for a moment that the range of is invariant under the operation of , i.e., for . Then, , since is the orthogonal projector onto . Thus, under this assumption the desired equation does hold, and if we assume that the range of is almost invariant under the action of then the equation is still a good approximation.
As a final point, if we want to compute the time evolution of a quantum system defined by a Hamiltonian , we want to compute for some value of . We note that as the decomposition is invariant with respect to (non-zero) scalar multiples, we may modify to give
For more details on related methods see the following literature:
E. Gallopoulos, Y. Saad "On the parallel solution of parabolic equations." Proceedings of the 3rd international conference on Supercomputing, 1989 17-28. doi: 10.1145/318789.318793
E. Gallopoulos, Y. Saad "Efficient solution of parabolic equations by Krylov approximation methods." SIAM journal on scientific and statistical computing 13(5) 1992: 1236-1264. doi: 10.1137/0913071
Y. Saad, "Analysis of some Krylov subspace approximations to the matrix exponential operator." SIAM Journal on Numerical Analysis 29(1) 1992: 209-228. doi: 10.1137/0729014
Implementation
Protocols
HQS Quantum Solver makes extensive use of Protocols, which means that it is easy to write code interfacing with Quantum Solver. Whenever you encounter a protocol class in the type hints of a function or method, the protocol class defines which methods and properties the corresponding argument object is expected to have and the corresponding return type is going to provide, respectively.
For example, the
evolve_using_krylov
function
expects a hamiltonian
argument which adheres to the
SimpleOperatorProtocol
.
To be allowed to pass an object as hamiltonian
, the object just needs
to implement all the methods and properties listed in
SimpleOperatorProtocol
,
which is in contrast to
abstract base classes,
where the object would need to be explicitly derived from the abstract base
class.
StateMaps
HQS Quantum Solver is able to exploit various conserved properties by
implementing, so-called, StateMaps
. A StateMap
provides a list of the Fock
states that are used to build an operator; it defines the basis set of our
Hilbert space. By specifically generating Hamiltonians and generic operators,
with custom StateMaps
, you can efficiently exploit these properties. This is
typically done automatically when specifying things like conserved particle
number, by mod_N = 0
, or conserved Sz
quantum number, by mod_Sz = 0
.
Dyadic representation
We also provide a special kind of operator that exploits the so-called dyadic
representation. Specifically, this methodology can be used for systems where
the state exists in a product of spaces, i.e., and the operators acting on it are either diagonal in the extended
space or may be described by Kronecker sums/products of operators in the
individual spaces, implemented by
DyadicOperatorSum
and
DyadicOperatorProduct
,
respectively.
We demonstrate the usefulness of this concept by evaluating the Hubbard Model. Starting with a spinful fermion model, we assume that the wavefunction can be expressed as a Kronecker product in the spin-up and spin-down degrees of freedom .
To do this we use a well-known matrix vectorization trick in reverse, let denote the so-called row-major vectorization operator acting on the matrix , then the Kronecker product has the property that
Similarly, if the operator is a Kronecker sum, Using the first property, we get that and and therefore, combining with the second property, This expression is the representation that is preferable to directly representing the operator on the product space, because it requires less storage and fewer operations to execute when applying the operator.